Cryogels are porous, sponge-like materials comprising an interconnected network of macropores, which are of great importance for applications such as 3D scaffolds for tissue culture, filters, or membranes. The application of cryogels is dependent on the size of their interconnected pores. In this project, various cryogels were prepared and pores were analyzed with automated methods.
A comprehensive image analysis process utilizing both classical thresholding and deep learning (DL) with Cellpose was developed.
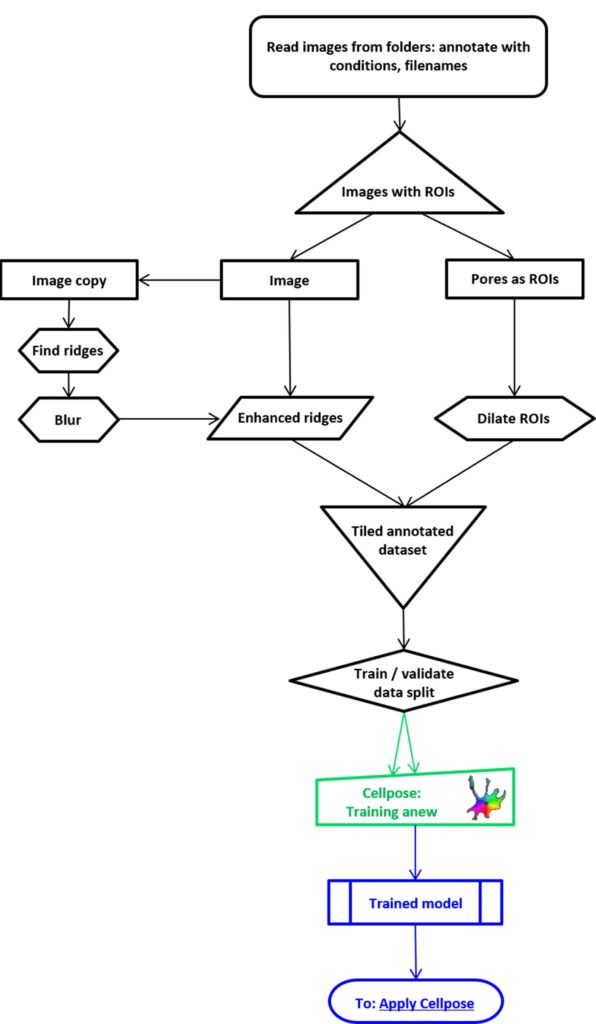
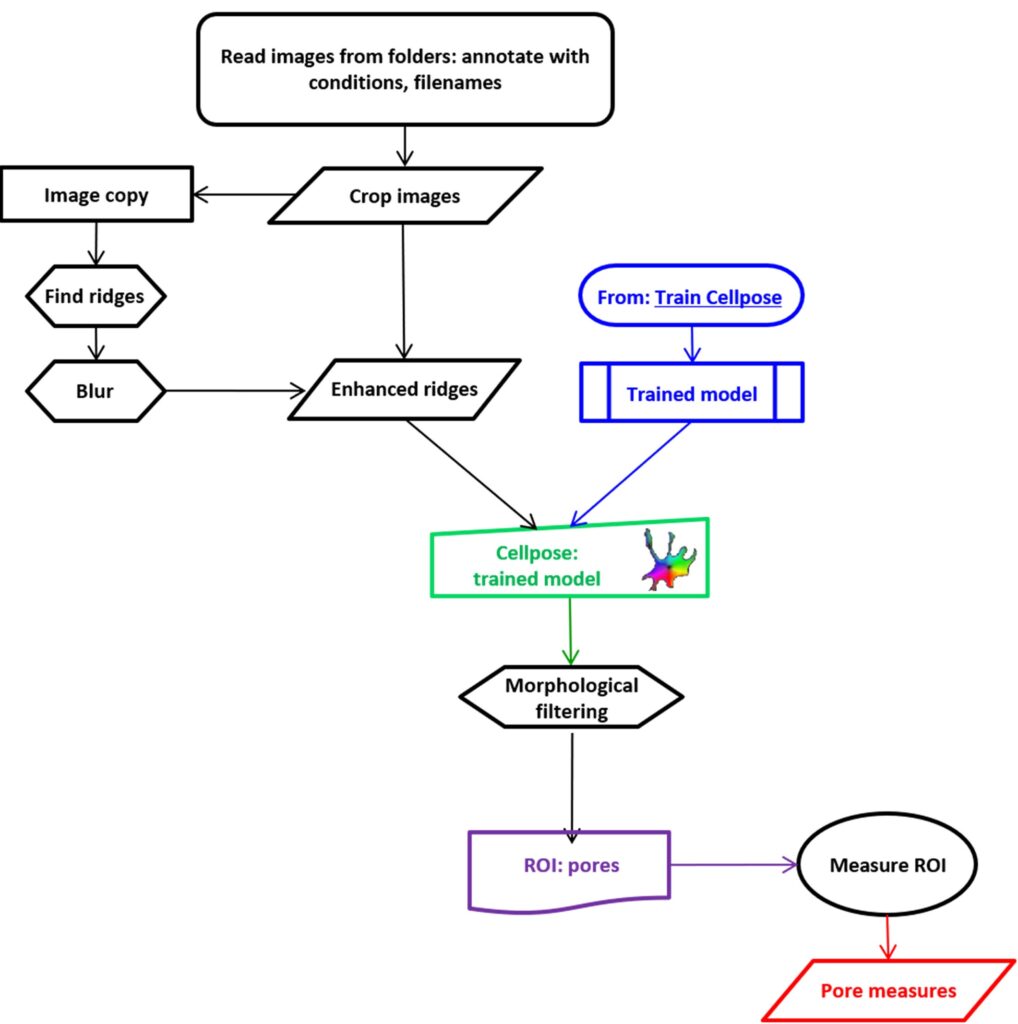
The analysis of electron micrographs was conducted using JIPipe, a software that automates the measurement of pore morphology. This was achieved by integrating classical global and local thresholding methods with a deep learning approach that employed the Cellpose algorithm with transfer learning, thereby enhancing the recognition of pores. The manual annotation and the training of two Cellpose models enabled the precise segmentation and quantification of pore characteristics. This demonstrated that the DL method is more accurate than manual and mercury intrusion porosimetry measurements in providing detailed morphological parameters.
The cryogel gels displayed robust reliability, with a high proportion of values obtained from manual and automated measurements showing minimal discrepancy. Additionally, cryogels prepared by the conventional method exhibited notable reproducibility.
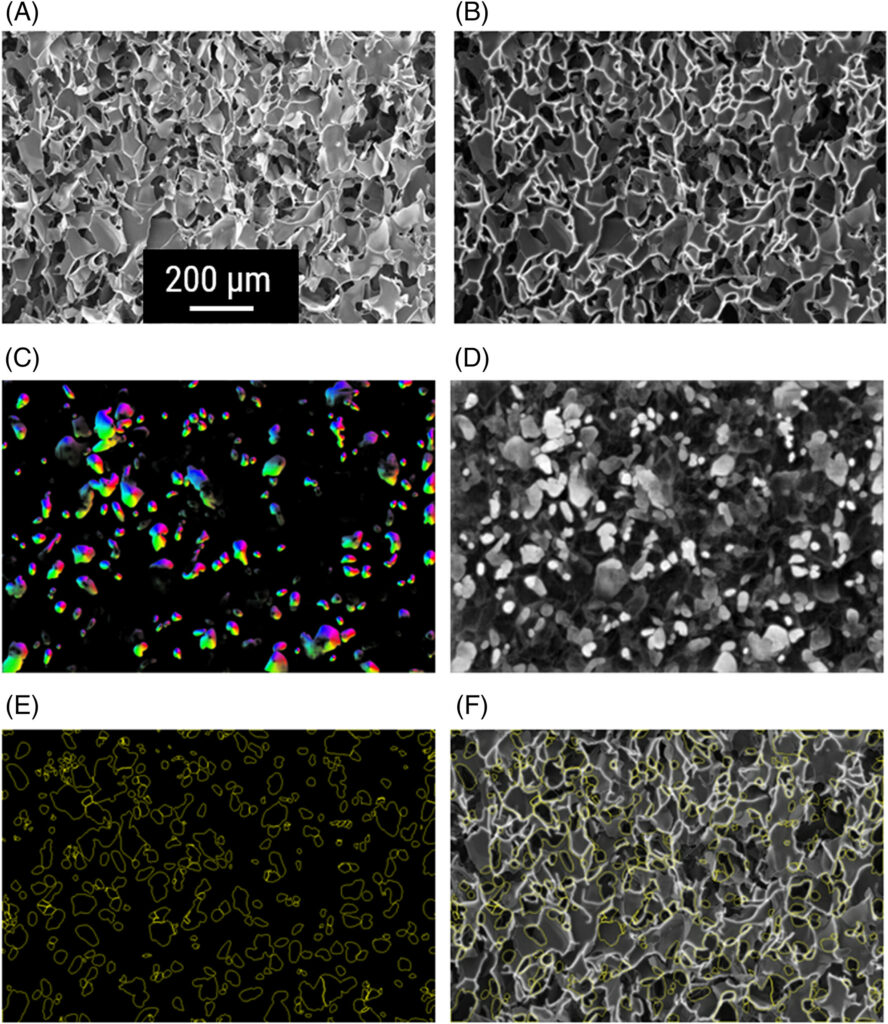
The deep learning-based algorithms developed in this project offer a significant advantage over classical and manual segmentation techniques for characterising objects with diverse shapes, sizes, and grey values. As evidenced by our transfer learning approach for identifying smaller pores, these algorithms demonstrate the capacity to adapt readily to new object types. The pipeline was effective in segmenting individual pores, thereby facilitating a detailed morphological analysis of objects and entire samples. In comparison to manual techniques, our automated methodology yields objective outcomes that are consistent with expert analysis in a considerably shorter time frame. Our algorithmic approach demonstrated that the syringe-based method reliably produces cryogels with consistent pore sizes.
Experimenal Collaborators
Laboratory of Organic Chemistry and Macromolecular Chemistry (IOMC) at the Friedrich Schiller University in Jena
Jena Center for Soft Matter (JCSM) at the Friedrich Schiller University in Jena