This project addresses antibiotic resistance by developing a high-throughput platform for rapid antibiotic susceptibility testing. The platform integrates microfluidic droplet encapsulation of individual bacterial cells, (ii) 2D angle-resolved light scattering (ARS) for label-free imaging, and (iii) deep learning algorithms to analyze microbial growth dynamics.
This project used advanced microfluidic and AI technology for rapid and reliable antibiotic susceptibility testing in just two hours.
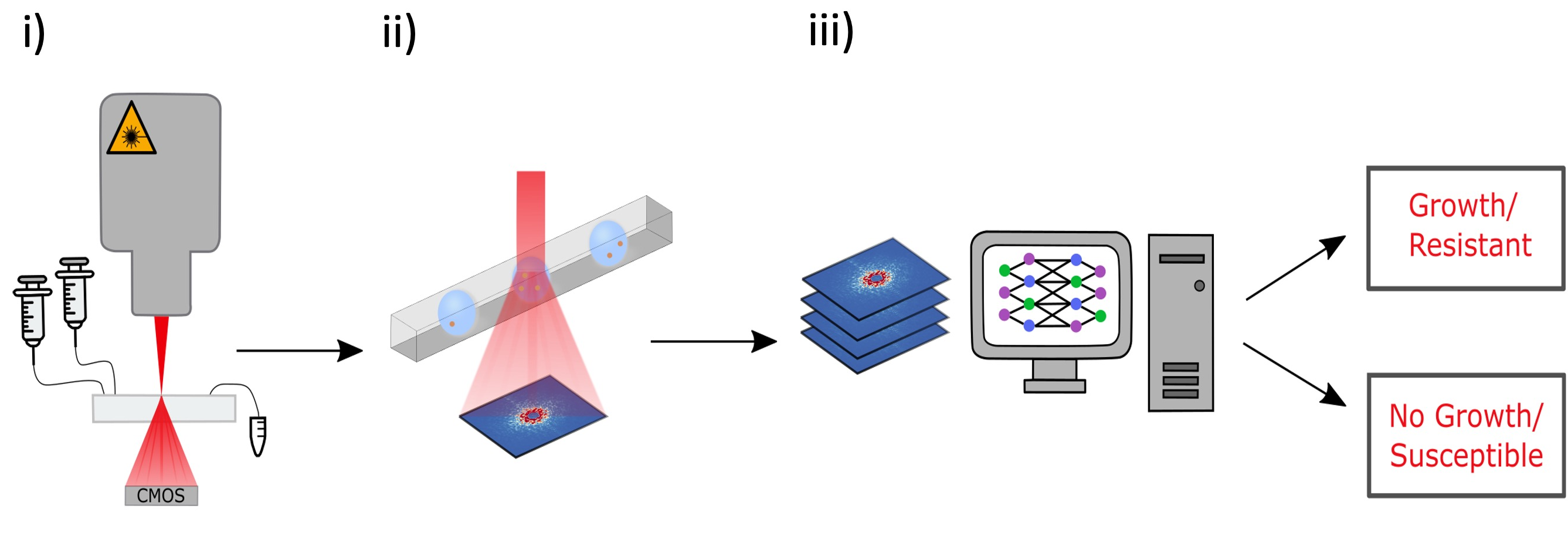
The analysis process begins with laser-based ARS imaging of picoliter-sized droplets within a microfluidic chip. Different exposure times (800, 1500, 2000 µs) were employed for imaging droplets, and principal component analysis (PCA) was utilized to visualize distinct OD clusters. EfficientNetV2 models were specifically used for feature extraction and OD prediction to enhance the accuracy of bacterial growth detection.
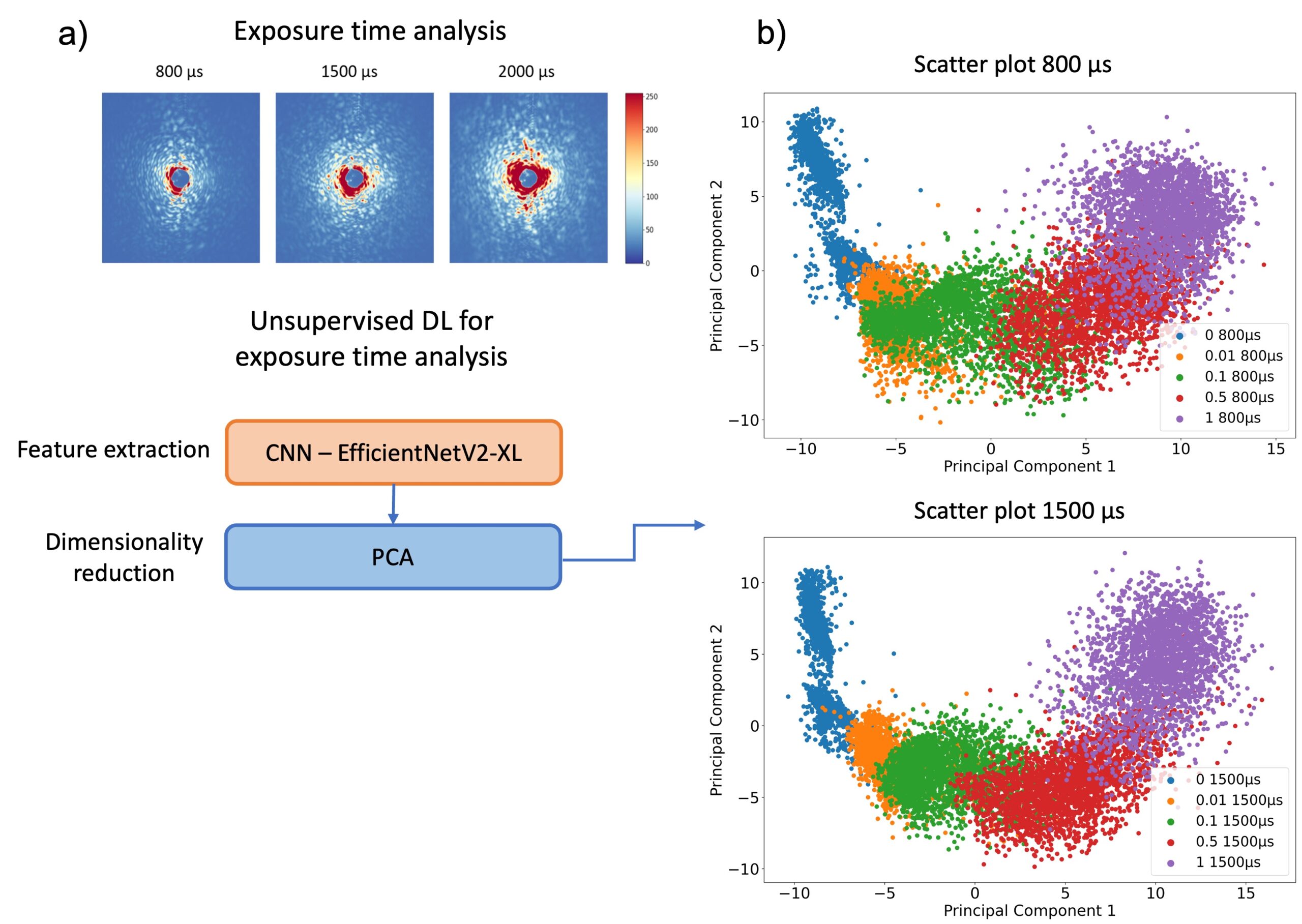
The ARS images are subsequently analyzed using convolutional neural networks (CNNs) to predict optical density (OD), thereby determining bacterial growth. This approach facilitates rapid and reliable antibiotic susceptibility testing, supporting high-throughput screening and offering improved sensitivity compared to traditional method.
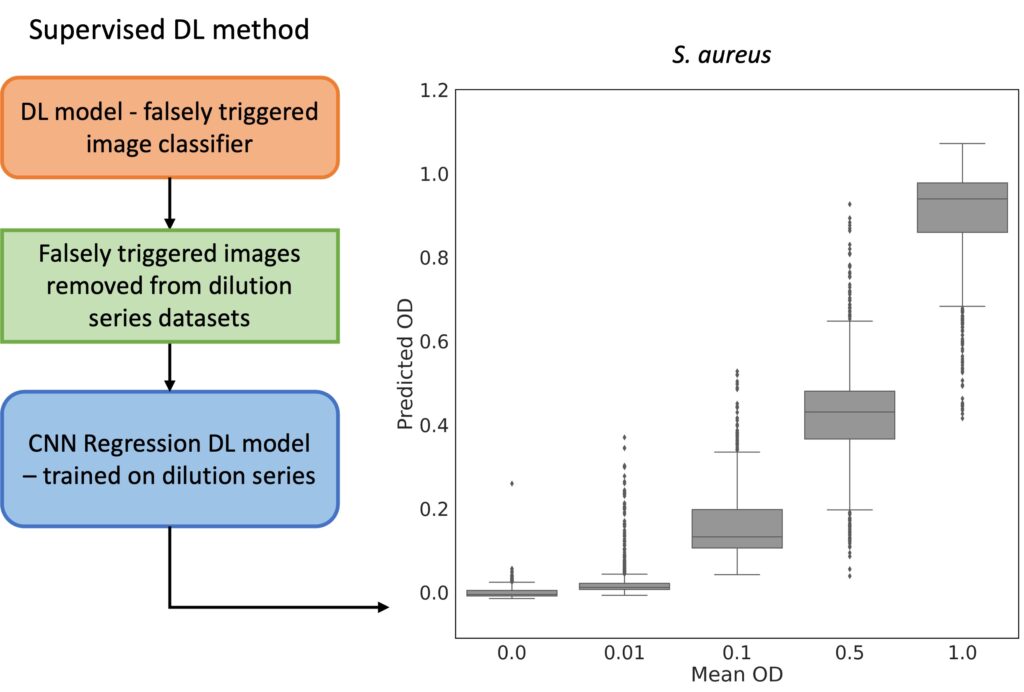
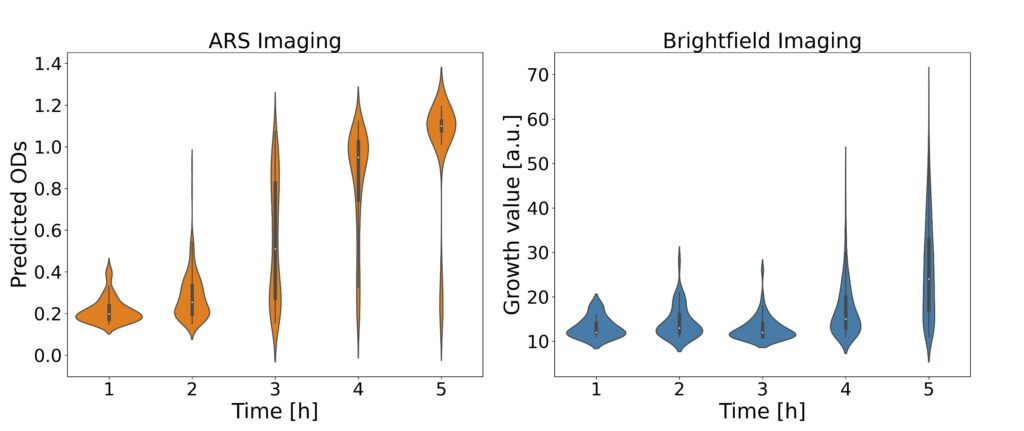
The platform employs microfluidic droplet encapsulation, angle-resolved light scattering (ARS), and deep learning algorithms to detect bacterial growth and antibiotic susceptibility in a time frame of one to two hours, a significant improvement over our previous methods. We demonstrate the efficacy of the method indicating the potential for transformative improvements in clinical diagnostics.
Experimental Collaborators
HKI Bio Pilot Plant at the Leibniz-HKI in Jena, Germany.