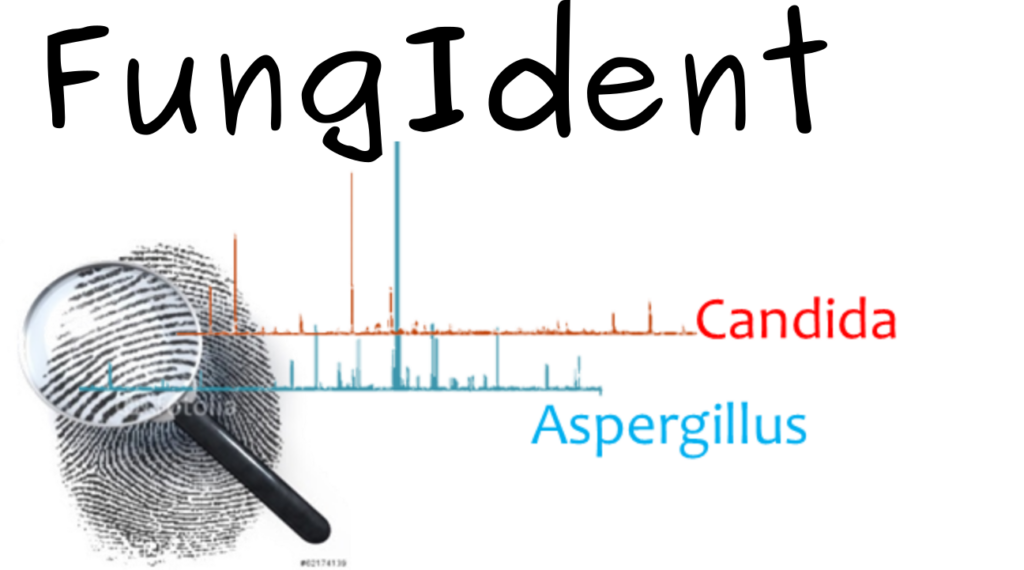
Experimental data
If the human immune system is weakened, fungal infections can lead to a life-threatening condition in humans. In order to determine the type of fungus, the pathologist has to cut out a piece of tissue from the organ to be examined. The diagnosis is based on manually inspection of histologically stained tissue slices. This is a time consuming and labor intensive task where the identification is highly dependent on the subjective judgment of the individual pathologist. Regardless of which images are used for the pathologist’s examination, the pathologist needs a lot of time to examine the entire tissue due to the far above-average size of the image. An alternative and relatively new method is MALDI-imaging (matrix-assisted laser desorption ionization), in which the mass spectrum of the tissue is measured without destroying the sample.
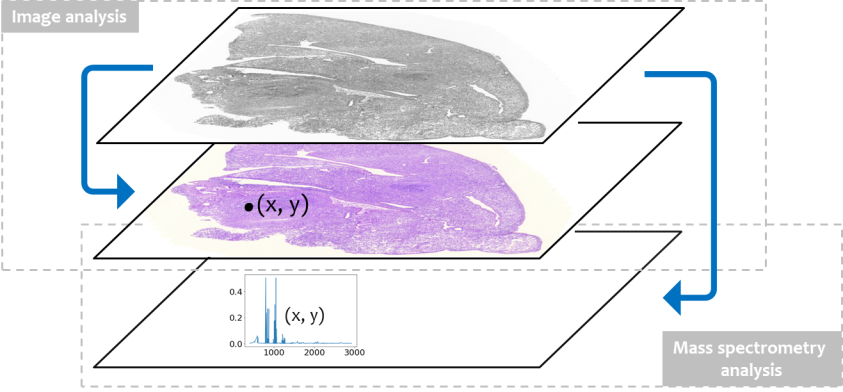
Correlative microscopy – spectroscopy approach
FungIdent is a correlative approach that accelerates diagnosis by guided analysis of spectra from MALDI images at pre-selected points in stained tissue sections, allowing identification of the molecular signature of each fungal genus.
Experimental Collaborators
- AG MALDI Imaging at the University Hospital Jena, Germany
- Institute for Hygiene and Microbiology at the Julius-Maximilians University Würzburg in Jena, Germany
Automatic Segmentation and Classification of Fungal-Infected Tissue using Deep Learning
Pixel-wise segmentation of histological tissue slices
A common method of highlighting the fungi is to stain the entire histological sample slices. Here we learn a convolutional neuronal network (CNN) that is able to learn features of images to distinguish between normal tissue and fungal-infected tissue independent of various environmental conditions such as the used staining method (Periodic acid-Schiff (PAS) staining and Silver staining), the organisms of human or mouse, the organ (e.g. lung, kidney, oral cavity, …) and the corresponding fungal genus (Aspergillus, Candida, Mucorales).
We provide a framework called SegNet that is able to examine these huge images and automatically segment and classify different types of fungi on different types of organs.
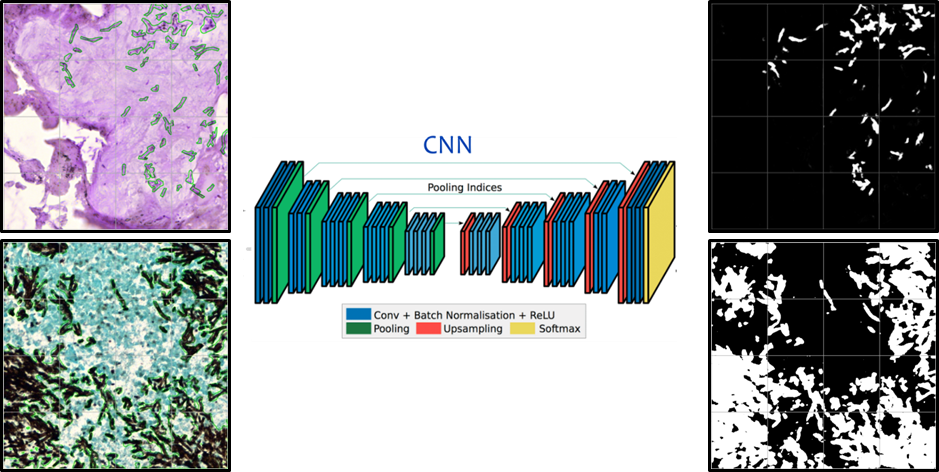
SegNet: A Deep Convolutional Encoder-Decoder Architecture for Image Segmentation
A practical deep fully convolutional neural network architecture for semantic pixel-wise segmentation termed SegNet, which is capable of detecting fungus under various environmental conditions such as staining.
Histological staining offers only morphological information, like color and shape. This makes it difficult for the pathologist to identify the exact species of the pathogen from a visual point of view. For clearly identification and resultant therapy strategies the state of the art diagnosis are insufficient. A fast and correct identification of a pathogen on a molecular level is a crucial point to ensure an efficient treatment of a patient.
Identification of the specific fungal genus based on spectra data
An alternative and relatively new method is MALDI – mass spectra imaging (MSI), in which mass spectrometry of the tissue is obtained at multiple positions to acquire an “image” of the molecular composition. We will present a framework to automate the process of fungal identification in histologically stained tissue slices with the goal of combining this information with the MALDI-MSI spectra of the same tissue sample. We have histologically stained slices of tissue from humans that are infected by different fungal genus, including Aspergillus, Candida and Mucorales. By co-registration of the histological images and the MALD-MSI results, the positions of the individual mass spectra within the matrix can be located and used as labels.
A second CNN of the analysis of the MALDI-MSI data called ResNet will be combined with the output of the optical images. We can then identify species and the spatial location for various kinds of fungal-pathogens based on this second deep learning approach. To extract the information of a certain pattern profile in the mass spectra for a certain genus, we have a deeper look at the learned features of the CNN.
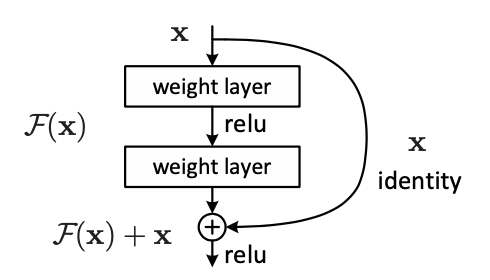
Identification of the single fungal genus
The ResNet is able to learn very fine features while skipping redundant features.
The deep learning framework provides a potential new diagnostic method to speed-up a time-consume process for a pathologist for examine fungal-infections.