The amount of fat in muscle tissue affects how well a muscle works. To distinguish fat from muscle tissue, ORO staining is utilized, as fat yields in a highly saturated red tone with the surrounding muscle tissue in a purple-blue to pale pink tone. Additionally, experts can distinguish fat areas, as well as fatless holes and cracks, from an easy-to-apply and cost-effective standard H&E-stained muscle section.
In this project, quantitative imaging with deep learning is employed to evaluate histological muscle samples with regard to their fat amount.
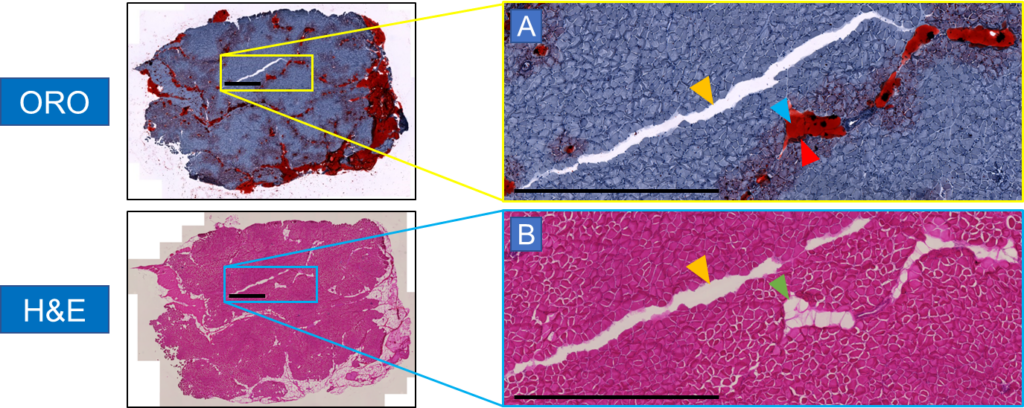
In order to know exactly what is really fat, our experimental collaborators manually annotated the H&E images of the muscle sections in ImageJ with the help of adjacent samples stained with ORO serving as additional references for a precise annotation. The quality of these manual annotations must be of the highest standard, which typically requires a workload of several hours to days.
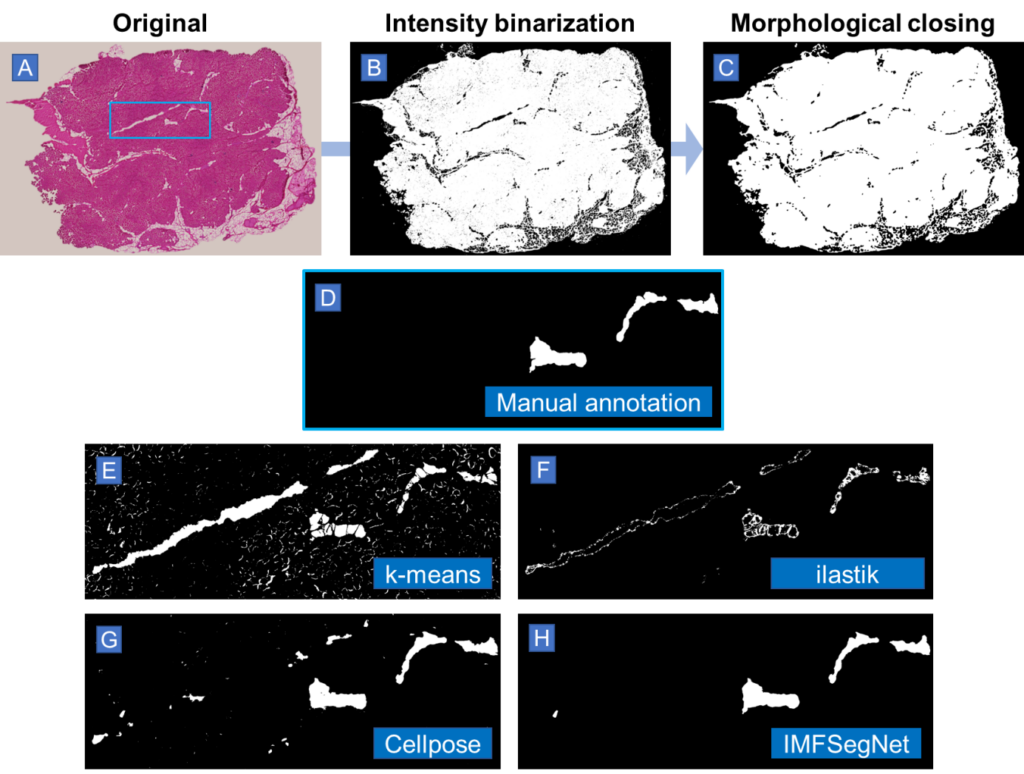
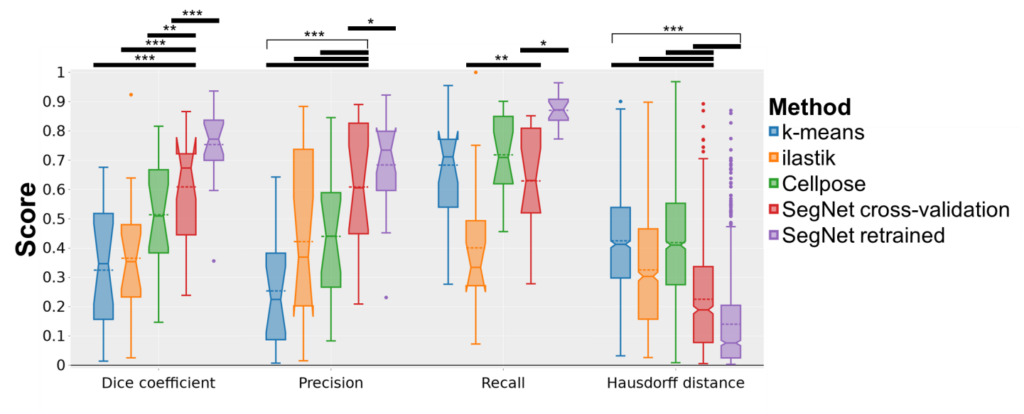
A variety of automated image analysis approaches were evaluated for the purpose of segmenting intramuscular fat (IMF), including intensity-based k-means clustering, the machine learning-based segmentation tool ilastik, the deep learning-based tool Cellpose, the convolutional neural network SegNet in the scope of a cross-validation, and the SegNet model retrained on all the available data. These techniques were compared to manual annotations from experts in order to assess their efficacy. The key performance indicators employed for this analysis included the Dice coefficient, precision, recall, Pearson’s correlation coefficient, and Hausdorff distance.
The results demonstrated that traditional methods such as k-means clustering and ilastik exhibited limitations in terms of accuracy. In contrast, deep learning approaches, particularly the retrained SegNet model (IMFSegNet), demonstrated superior performance, achieving balanced precision and recall, high correlation with manual annotations, and lower Hausdorff distances. Furthermore, IMFSegNet exhibited robustness across varied staining intensities, making it a cost-effective and reliable tool for analysing IMF in muscle tissues.
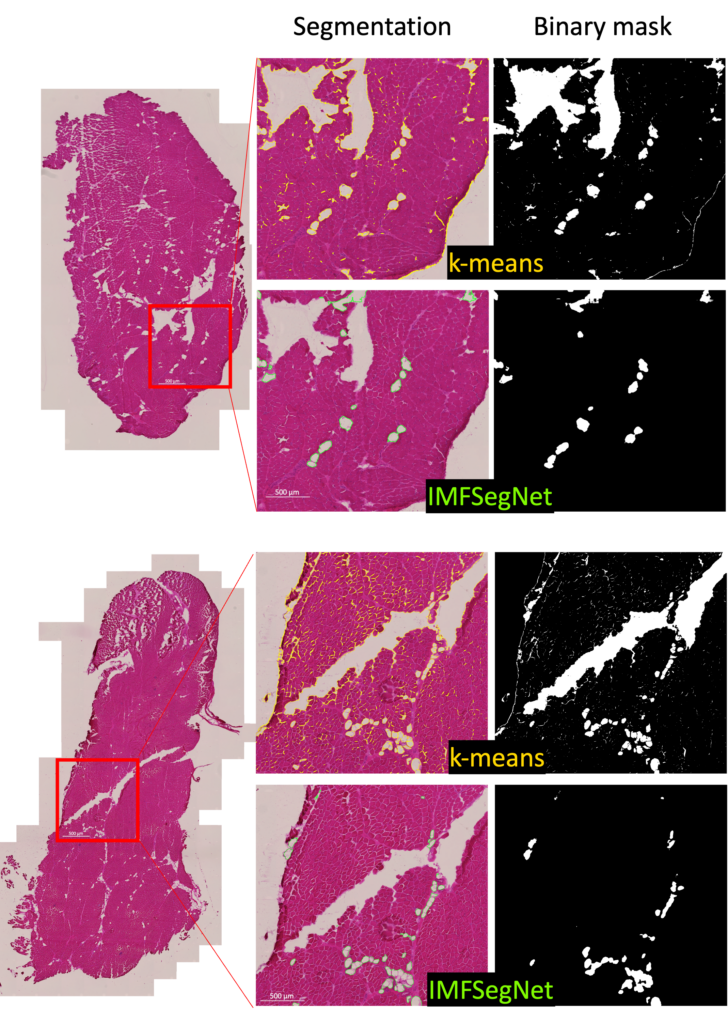
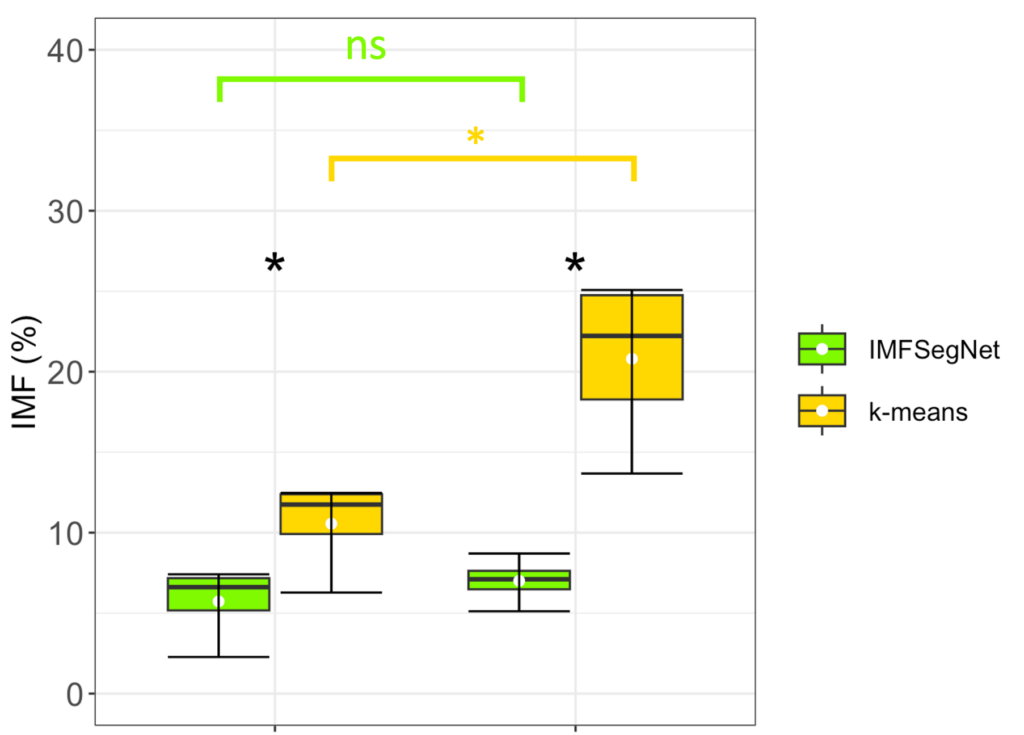
To demonstrate the strength of IMFSegNet, the method was tested with a real data set and compared to k-means clustering. The standard deviation of the k-means clustering was found to be significantly larger, indicating greater variation and lower accuracy compared to the IMFSegNet method. The mean fat amount was found to be significantly higher with k-means clustering than with IMFSegNet, which demonstrates the tendency of k-means to overdetection. The use of IMFSegNet for automated analysis was found to be faster and more accurate than manual annotation and k-means clustering. This approach provides a reliable method for large-scale quantitative imaging, supporting the development and clinical implementation of such techniques.
Experimental Collaborators
- Institute of Zoology and Evolutionary Research of Friedrich-Schiller-University Jena, Germany