The Multi-Model Simulator (MuMoSim) project aims to advance modeling in infection research to the next level by introducing a novel quantitative and predictive simulation tool. The standout features of the framework are based on the self-consistent integration of various modeling approaches within a single framework. As a result, MuMoSim can provide reliable quantitative predictions with only limited experimental data, with the flexibility to include more experimental data of varying complexity. Combined with an experimental setup based on human whole-blood infection assay, MuMoSim presents a coherent, next-generation modeling tool to quantify innate immune effector mechanisms for difficult-to-treat infections and ultimately predict treatment strategies.
The Multi-Model Simulator is a novel quantitative and predictive simulation tool.
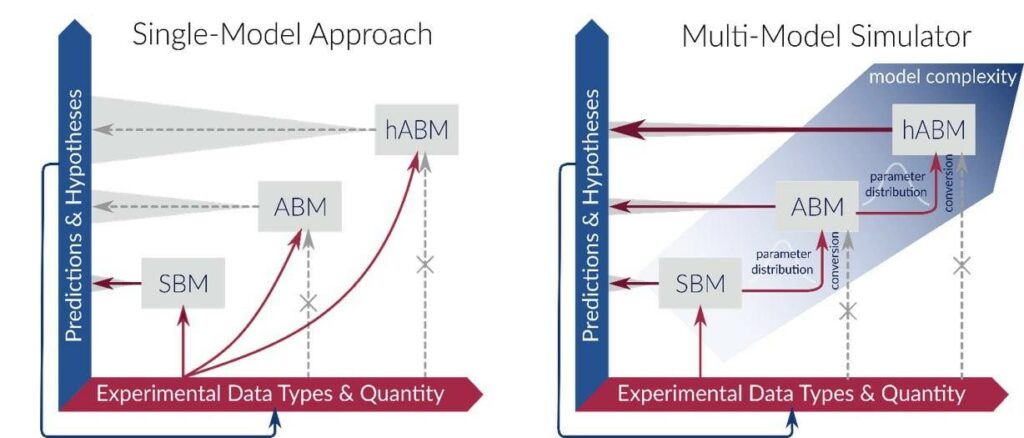
Originally based on the bottom-up approach, MuMoSim is capable of modeling (i) well-mixed systems of interacting cells using state-based modeling (SBM), (ii) analogous but spatially heterogeneous systems by means of agent-based modeling (ABM), and (iii) spatial systems with interacting cells and molecular concentrations via hybrid ABM (hABM). Within the framework, the parameters are estimated by means of Approximate Bayesian Computation, and posterior distributions for models of lower complexity are used as priors for the models of higher complexity.
Experimental Collaborators
Fungal Septomics at the Leibniz-HKI in Jena, Germany